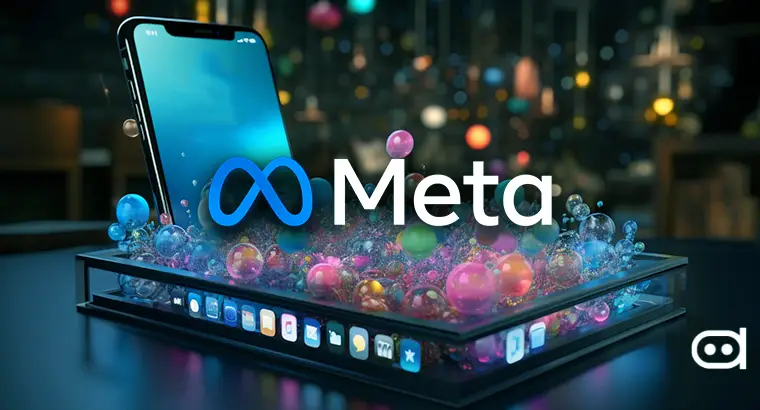
Meta has announced the launch of Quantized Llama 3.2 models. The objective is to offer developers more convenience with reduced memory usage. It is estimated that the deployment of Llama 3.2 can reduce memory usage by almost 41% while boosting its speed by around 2-4 times.
Meta has also achieved an average reduction in model size of 56% by leveraging two techniques, namely Quantization Aware Training and SpinQuant. Llama 3.2 1B and 3B were open-sourced last month at Connect 2024. Meta is now building on it to carry forward a legacy.
With Llama 3.2, developers can build without digging deep into heavy requirements about resources and expertise. It is compatible with mobile phones and works its way around shorter runtime memory. Meta has prioritized short-context applications up to 8k to support operations on resource-constrained devices, which refers to mobile devices.
Llama 3.2 essentially helps deploy quantized models to more CPUs with higher privacy levels and faster deployment speeds.
A Quantization-Aware Training tool with LoRA adapters helps optimize performance in environments where precision is hard to achieve. SpinQuent helps determine a perfect compression combination without affecting the quality of the work. It has worked with industry leaders to make the models available on Qualcomm and MediaTek SoCs with Arm CPUs.
Meta’s quantization set-up is paired with PyTorch’s ExecuTorch inference framework and Arm CPU backend. It considers prefilling or decoding speed and memory footprint. The scheme has three parts: quantization of the linear layer, classification layer, and final employment.
Quantizing linear layers for weights and 8-bit per-token dynamic quantization is handy for activations. Linear layers are practically quantized to a 4-bit Group-wise scheme with a group size of 32.
The classification layer is then quantized to 8-bit per channel for weight and per token dynamic for activation. The 8-bit per-channel quantization is effective for embedding only.
Meta has expressed satisfaction with its results so far. In the announcement, it said the growth has been 10 times more than estimated, making it the standard for responsible innovation.
Llama is competing with other players in the market to lead instead of mere survival. Its stand is based on three pillars: modifiability, openness, and cost efficiency. Llama is now being architected to be available on Hugging Face and llama.com.
This comes days after Meta briefed about Llama being used by Untukmu.AI to protect the privacy of its customers. The Indonesian platform integrated Llama to back design a semi-decentralized personal assistant.
The goal of Untikmu is to ensure that customers are helped at every turn without the company having to examine their data. Llama was a perfect fit for its balance between the quality of output and the efficiency of resources.
Meta is confident about Llama and looks forward to building Llama 3.2 models further for enhanced performance.
Source: https://ai.meta.com/blog/meta-llama-quantized-lightweight-models/
Latest Stories:
AI Sales Platform Attention Secures $14M to Transform Sales Teams
NayaOne and NVIDIA Partner to Fast-Track GenAI in Financial Services
Machine Learning Powers Next Leap in Circular RNA Gene Therapy