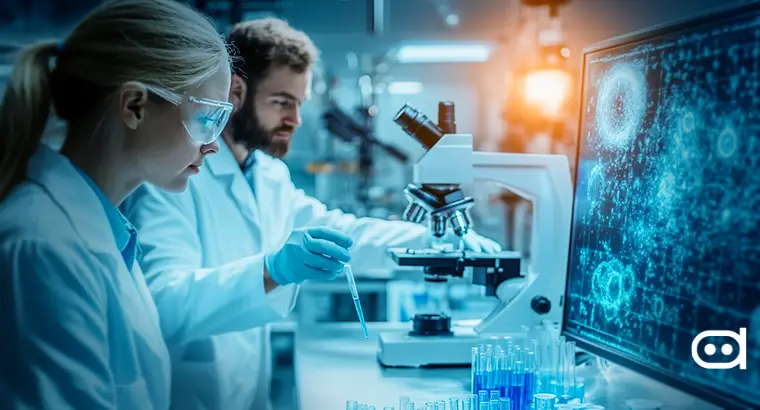
In The Jetsons, a classic cartoon, a robotic maid named Rosie, became popular. She switches between various household chores, from cooking dinner to vacuuming and cleaning the house to taking the trash out. However, the reality is very different, and training a robot for general purposes still remains a big challenge.
Engineers typically collect information specific to certain tasks and robots that they employ to train them in a controlled environment. But the major challenge lies in collecting data, which remains time-consuming and costly.
Moreover, robots are most likely to find it difficult to adapt to various environments that they have never seen before.
MIT researchers have designed a more versatile technique to train general-purpose robots more effectively. The technique involves combining massive heterogeneous data from various sources into a single system that teaches robots different types of tasks.
By aligning information from various domains, such as real robots and simulation, the method, along with multiple modalities such as robotic arm position and quarters and vision sensors, is built into a language that generative AI models can process.
The combination of enormous amounts of data can help train robots to perform different tasks without requiring training from scratch every time. The new method could prove to be more cost-effective and faster than any traditional techniques we have seen, as it will require less task-specific information.
Added to that, the method has outperformed by over 20% in real-world experiments and simulations. An EECS (electrical engineering and computer science) graduate student, Lirui Wang, mentioned in his paper on the new technique that people claim that in robotics, there is not enough training information, but the real problem lies in the fact that the information comes from different domains, robot hardware, and modalities which makes it more challenging.
Wang works to train robots by putting them together.
The robotic “policy” considers sensor observations such as camera images and proprioceptive measurements which track the position and speed of the robotic arm and determine where and how to move.
Typically, the policies are trained with imitation learning, which means humans demonstrate the actions and teleoperate with the robot to generate information that is put into the AI model. Since the method requires very small amounts of task-specific information, robots might fail when the task or environment changes.
For a better and more effective approach, Wang collaborates with others to draw inspiration from GPT-4 and other large language models. The researchers at MIT designed the heterogeneous sprained pretrained transformers (HPT), which unify information from varied domains and modalities.
However, the biggest challenge of designing HPT lies in developing a huge data set for pretraining the transformer, which includes 52 data sets with over 200,000 robot trajectories in four different categories, including simulation and human demo videos.
However, after testing HPT, it improved the robotic performance by 20% on real-world simulations and tasks compared to the previous model, which involved training from scratch. The Toyota Research Institute and the Amazon Greater Boston Tech Initiative fund the entire work.
Source:
https://news.mit.edu/2024/training-general-purpose-robots-faster-better-1028
Latest Stories:
Hong Kong Embraces Blockchain and AI for Fintech Growth
AI Adoption Urged for Lawyers at Queensland Government Conference
Robotics and AI Revolutionize Science Labs for Faster Breakthroughs