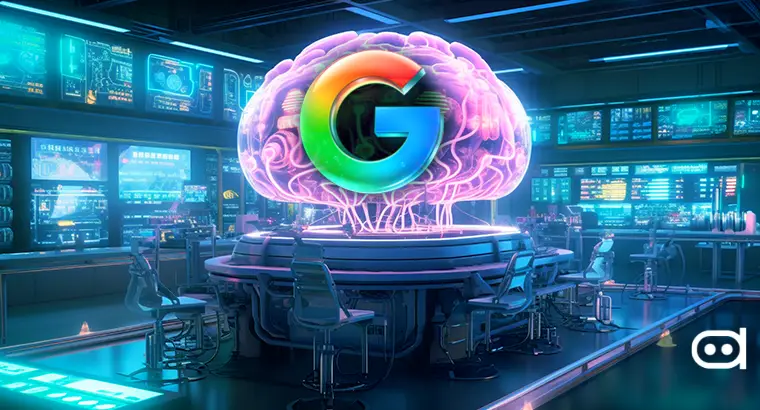
On 26 October 2024, Google Cloud announced major updates to improve training and influence performances on AI Hypercomputer software, as well as improved resiliency and a centralized resource hub.
Google Cloud’s supercomputing architecture, AI Hypercomputer, combines open software, AI-optimized hardware, and consumption models to improve productivity and efficiency.
The open software layer on AI Hypercomputer will support top ML Frameworks, provide workload optimizations, and provide a reference implementation for enhancing time-to-value for certain use cases.
To make the open software stack accessible to practitioners and developers, Google Cloud will introduce the AI Hypercomputer GitHub Organization, where users will explore reference implementations like MaxDiffusion and MaxText, various orchestration tools like PK, and performance systems for Google Cloud’s GPUs.
MaxText will now support A3 Mega VMs backed by NVIDIA H100 Tensor core GPUs, offering a 2X improvement in GPU-to-GPU bandwidth across these VMs.
Mega VM-powered MaxText will now deliver linear scaling and achieve additional hardware, utilization, and acceleration.
For most MoEs (mixture of experts) use cases, consistent utilization of limited expert resources is useful, but for specific applications, having more experts in designing richer responses can be of greater importance.
To provide greater flexibility, MaxTest has been expanded to include no-cap and capped MoE implementation so users can choose what best suits their model architecture. While a capped MoE model offers predictable performance, a no-cap model dynamically allocates all the resources for optimum efficiency.
Pallas kernels have been made open-source for further training acceleration by optimizing block sparse matrix multiplication. You can now use kernels with both JAX and PyTorch and enjoy high-performance blocks for MoE model training.
AI Hypercomputer is slowly building blocks for AI’s next generation by pushing the limits for model training and inference and enhancing accessibility via key resource repositories. AI practitioners in the future will be seamlessly scaling from concept building to production without any burden put on by infrastructure limitations.
Check out Google Cloud’s official website to learn more about the latest software updates and resources on AI Hypercomputer, including an accelerated processing kit, optimized recipes, and reference implementations.
Source:
https://cloud.google.com/blog/products/compute/updates-to-ai-hypercomputer-software-stack
Latest Stories:
Hong Kong Embraces Blockchain and AI for Fintech Growth
Robotics and AI Revolutionize Science Labs for Faster Breakthroughs