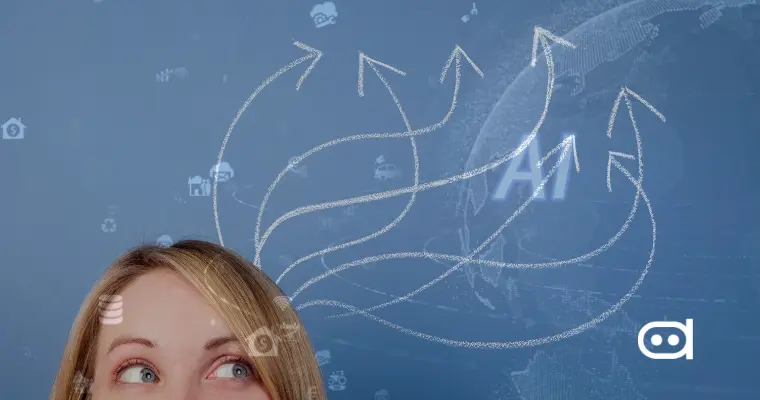
Since artificial intelligence has been created using human knowledge and emotions, there is also a vast chance for AI bias in HR. AI has been implemented into the hiring process for various job roles to minimize time and improve efficiency. Overcoming AI bias is a skill that the recruiter must be aware of and this article sheds light on the strategies. It provides in-depth information regarding how to achieve bias-free hiring.
Understanding AI Bias in HR Automation
AI tools for HR automation are directly impacted by the potential for AI to detect bias in data and information provided to it or in the algorithm itself. Many factors, including algorithmic impact, imbalanced data, and social biases in the data, might lead to hiring prejudice when utilizing AI. Algorithmic bias happens when the AI has previously been fed information into the algorithm regarding which kind of candidates are to be filtered. Sample bias happens when the real-world measurements and demographics are not correctly reflected in AI training data. It may result in issues like some communities being overrepresented or underrepresented due to AI bias mitigation.
AI Tools in HR Automation: Where Bias Can Arise?
Achieving bias-free AI in HR can be challenging, especially with the rising usage of AI tools. The large number of users can sometimes make the tool overworked, and some issues might arise due to the design of these tools.
AI-powered Resume Screening Tools
AI resume screening bias is one of the commonly occurring bias issues. These tools have been trained to undergo the hiring process, which might act as a bias toward favoring only a few candidates. One example of AI hiring bias is when Amazon found that its AI hiring tool favored male applicants; it terminated it, reflecting the gender gap in the workforce.
AI-driven Interview Tools
AI bias in recruitment happens during interviews, which were initially intended to increase recruiting efficiency and may unintentionally reinforce prejudices seen in their training data. For example, it has been shown that face recognition software is inaccurate in recognizing people with darker skin tones, which might result in prejudice. According to the documentary “Coded Bias,” which examines the societal consequences of biased algorithms, critics contend that these AI systems could serve to further pre-existing biases.
AI-based Performance Management Systems
AI-based performance management systems use artificial intelligence to analyze large volumes of data, including job outputs, communication patterns, and engagement indicators, to track, assess, and improve employee performance. To increase employee engagement and productivity, businesses like Centrical have created systems incorporating gamification, tailored microlearning, and AI-driven analytics.
AI-driven Employee Engagement & Retention Platforms
Use chatbots for employee engagement to improve work satisfaction and commitment. Artificial intelligence is used to evaluate employee data, forecast turnover risks, and customize interventions. This is one important type of bias in AI. Businesses such as Centrical utilize gamification and customized interventions. New developments include the advent of platforms like CloudFit, which provides individualized wellness programs to promote overall employee well-being, and ServiceNow’s acquisition of Moveworks, which aims to expand AI capabilities in employee assistance.
Strategies to Overcome AI Bias in HR Decision-Making
Certain strategies can overcome AI hiring bias. These strategies can also help to aid the decision-making process even more effectively. A few strategies are given below:
Enhancing Data Quality and Diversity
To reduce AI bias in decision-making, it is essential to improve the variety and quality of data. Reliable, representative datasets lower the possibility of biased results by ensuring AI systems learn from various events. AI systems may make more equitable judgments by combining various information and viewpoints, promoting fairness and confidence in technology-driven processes.
Algorithm Transparency and Explainability
In artificial intelligence (AI), algorithm explainability and transparency are essential for ensuring that automated judgments are comprehensible and responsible. Explainable AI (XAI) aims to solve the “black box” aspect of sophisticated models by making the internals of AI systems understandable to people.
Human-AI Collaboration for Fair Decision-Making
One example of this is the idea of Reciprocal Human Machine Learning (RHML), which encourages ongoing learning between AI systems and humans and ensures that both adapt and get better through feedback. Furthermore, interdisciplinary partnerships like the Partnership on AI seek to develop best practices that guarantee AI’s beneficial impact on international information ecosystems.
Implementing Bias Mitigation Techniques in AI Tools
Bias mitigation strategies must be incorporated into AI systems to guarantee equity and inclusion. To stop models from picking up biased tendencies, one of the main strategies is to select training data that is representative and varied. Additionally, imbalances may be found and fixed before model training by using bias detection methods during data preparation.
Regulatory Compliance & Ethical AI Practices in HR
The increasing integration of artificial intelligence (AI) into business operations has made it important for Human Resources (HR) to ensure ethical AI practices and regulatory compliance. To avoid biased outcomes, businesses that use automated systems for recruiting or promotions must do independent bias audits, as required by the New York City Bias Audit Law (Local Law 144), which goes into effect on July 5, 2023.
Future of Bias-Free AI in HR Automation
The future of being bias-free of AI in HR automation can be done by concentrating on candidates’ talents and skills despite giving AI information to filter candidates using predetermined data. To detect and address algorithmic drift, frequent bias audits are now an important procedure in which AI suggestions are routinely examined against diversity and inclusion categories.
Additionally, companies use AI frameworks that offer transparent recruiting, enabling human monitoring to identify biases. However, how well AI can implement this strategy relies on the data and algorithms’ design. Implementing measures that stop AI from reproducing preexisting biases is important to ensure fairness.
Summing Up
Organizations must prioritize data diversity, algorithm transparency, and human supervision to develop just and moral AI-driven recruiting procedures. Regular algorithmic audits are necessary to identify and address discriminatory behaviors when implementing strong bias-reduction measures. These audits should evaluate results for several demographic groupings and track advancements toward diversity objectives. Organizations must also set up explicit governance structures for their AI systems.