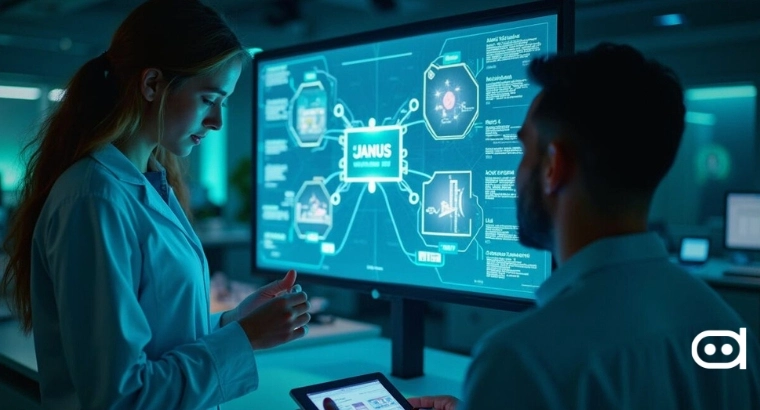
Hangzhou, China – 18th October 2024 – DeepSeek, a Chinese startup focused on artificial general intelligence (AGI), launched Janus, its novel autoregressive framework designed for multimodal understanding and generation tasks. Janus stands out by addressing limitations in earlier models by decoupling visual encoding into distinct pathways.
DeepSeek’s first multi-modal LLM will be available on Hugging Face, announced by Philipp Schmid, the Tech Lead and LLMs at HuggingFace, with a post on X:
First Multimodal Model from @deepseek_ai is now on @huggingface!
> Janus is a 1.3B unified MLLM, which decouples visual encoding for multimodal understanding and generation.
> Its based on DeepSeek-LLM-1.3b-base and SigLIP-L as the vision encoderhttps://t.co/jaOHLdG5Lh— Philipp Schmid (@_philschmid) October 18, 2024
While the visual encoding is separated for each task, Janus utilizes a single, unified transformer architecture for processing. By decoupling the visual encoding pathways, Janus resolves conflicts that visual encoders face: handling both understanding and generation tasks.
The launch of Janus represents the ability to integrate MLLMs seamlessly across various tasks, a significant improvement over its predecessors. It also leads to enhanced flexibility without sacrificing performance.
However, Janus not only surpasses previous models but also exceeds the performances of task-specific models. It improves the handling of multimodal inputs compared to older frameworks, making Janus a frontrunner among the next generation of unified multimodal models.
Janus is built on DeepSeek’s LLM-1.3b-base and is trained on approximately 500B text tokens. It also leverages SigLIP-L as the vision encoder, supporting image input resolutions of 384 x 384. This makes Janus a strong contender for potentially driving innovations in AI-powered content creation, multimedia analysis and more.
DeepSeek’s Janus positions itself as a leading solution in the evolving multimodal LLM landscape by offering decoupled visual pathways but retaining a unified transformer framework. Its flexibility without compromising performance will make it a popular tool among Hugging Face’s community and other AI developers.