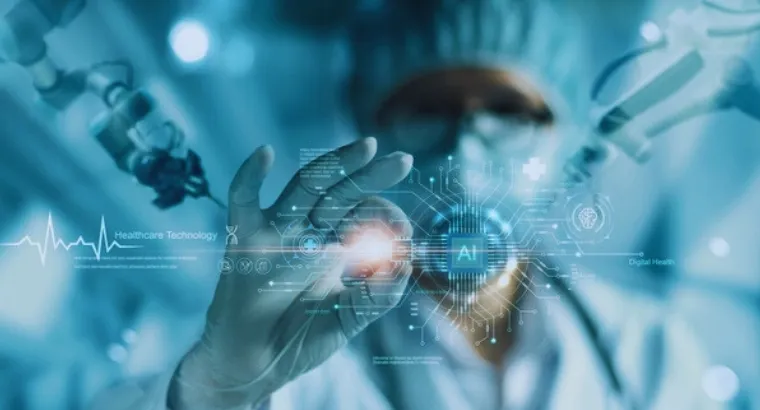
Technology is evolving to the extent that it is being applied to critical fields like medicine. Machine Learning, for one, has made an impact in this field by assisting medical professionals in monitoring patients’ health conditions and facilitating the process of diagnosing and treating ailments. A couple of research studies and professionals have shed light on how machine learning is coming to their rescue or how it can come to their rescue in the future.
New tools for medical research
One challenge that the medical department often faces is detecting MG-associated DCM, which is dilated cardiomyopathy. Early stages don’t necessarily demonstrate signs of detection despite the patient housing them in his/her body. When not diagnosed, the symptoms aggravate to weaken the muscles and cause easy fatigue.
The idea behind Machine Learning, here is to go for the potential diagnostic value of PI3K-interacting protein 1 (PIK3IP1) and midline 1 interacting protein1 (MID1IP1). Researchers have said that both play an important regulatory role in understanding the occurrence and development of multiple diseases. They added that more research and exploration are needed to uncover more data about factors contributing to MG-associated DCM. When understood, it will help uncover early detection for a more efficient treatment in the future.
As for MG-associated DCM, researchers have pointed out that their occurrence rate is relatively low, adding that if it ever occurs, then symptoms only aggravate to further damage the patient.
AI muscle takes center stage
Machine learning is pretty much a subfield of AI. When one mentions ML, they are bound to converge on at least a couple of aspects of AI in the conversation. It is imperative to understand this because the AI muscle here pertains to implementing ML in a manner in which medical professionals can assist their patients in monitoring conditions related to Parkinson’s disease. The work is in progress as the team intends to analyze a large data set of healthy and unhealthy bodies.
Wearable sensors that are inexpensive may improve access to care for Parkinson’s disease patients. A new study conducted by researchers and clinical collaborators at the University of Illinois Urbana-Champaign discovered that the accuracy of the results from these sensors can be improved using new machine-learning algorithms and a baseline of data from healthy older persons.
The subsequent development of a comparative model would provide ample foundation for experimentation and aid patients in obtaining precise information. This study aims to improve the accuracy of detecting changes in motor impairment in persons with Parkinson’s disease, according to Manuel Enrique Hernandez, the head of the study.
Patients otherwise have to undergo periodic assessments, which only creates difficulties for them. This is in addition to the existing challenges, which include a time-consuming process and the limited availability of in-person visits. The only challenge that ML presents in the segment is a lack of quantifiable measurements. The study is likely to resolve this as it progresses.
The expanding reach of Machine Learning
Medical professionals are in the initial stages of deploying machine learning to create distinctions between their tasks. For instance, the ML model has been trained to identify stem cells and adult muscle cells to create a real-time quantitative index that gives a numerical score to every cell.
They even found an unexpected result wherein the model demonstrated that differentiation is a gradual and decentralized process as cells do not progress together in the stages. If anything, they follow a different pattern of progress.
Another instance of the expanding reach of ML is Congressmen pursuing degrees in Machine Learning. The aim is to draft AI regulations better. The most recent entrant on the list is Don Beyer. He is a Virginia Democrat and a car dealership owner who has enrolled in the course at George Mason University. Their entry into academic life spans the segments of knowing the potential and risk behind the implementation of AI—thereby, ML.
Conclusion
Whether medical professionals or Democrats, the ultimate goal at the moment is to grasp the basics of ML-AI. A common objective is to eliminate its risks and magnify its opportunities.
One way to look at it is to monitor health conditions and develop new tools. A broader implementation rolled out in the future will paint a better picture. Lawmakers are practically exploring the field to navigate the rise of technology. The industry is currently anticipating the yet-to-be-determined outcome.