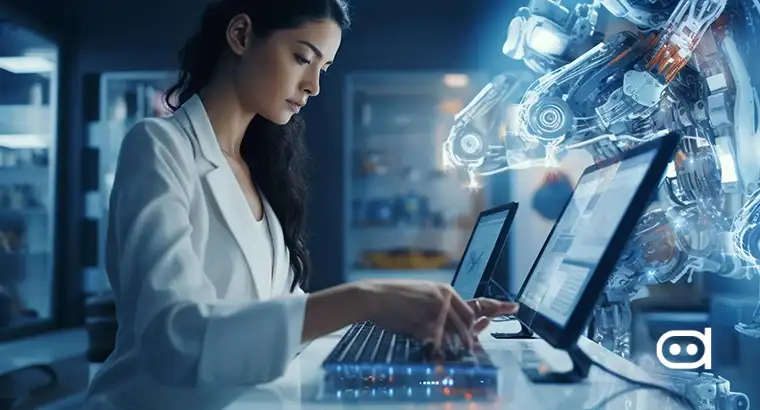
AI is transforming numerous sectors across the world, but a possibility of having AI bias in these systems is a concern. However, researchers from MIT have made huge strides in offering solution to this issue by designing an approach that neutralizes bias of the AI models while at the same time enhancing the capability of the AI models in making relevant predictions. This advancement offers a fresh opportunity of developing better strategies that could improve the fairness of artificial intelligence models.
Bias in artificial intelligence arises when the datasets employed in creating machine-learning algorithms contain biases that are replicated and perpetuated. This can lead to bias or incorrect decision making for some individuals especially those in the margin. Earlier approaches towards handling this issue have focused on a few means, such as creating subsets of the datasets that are similar in terms of the groups. But in doing this, one must sacrifice a lot of data often reducing the number of good samples to learn from by half or worse.
The solution proposed by the MIT team is an example of thinking about the given problem from a different angle and influencing specific indicators that affect the models’ errors for certain categories of citizens. Instead of seeking to balance the representation by eliminating points collectively, this technique selects and eliminates these undesirable points. Such an accurate approach minimizes the possibility of information loss while allowing the model to either retain or improve the basic accuracy level at the same time addressing the fairness concerns.
This approach is crucial when used to identify and eliminate unconscious prejudices in training data sets that do not contain specific attributes. While working with data in many real-world applications, such information referring to race, gender, or socio-economic status is not usually provided as comprehensive labels that would reveal bias within a dataset. This new approach uses techniques that reveal these hidden biases, making the model more fair in its prediction even where such data is unknown.
This progress is particularly crucial for domains that are highly sensitive to fairness. For example, if an AI system is trained on a dataset that does not include minorities, the AI model may produce tests that inaccurately diagnose or suggest different treatment regimens for people of color, thereby endangering their lives. The approach that was developed by the MIT researchers helps overcome this problem by introducing less bias while remaining as precise as it should be, which in turn means that there are possibilities for refined and less prejudiced AI in vulnerable scenarios to take place.
The implication of this study is not limited to the medical field only. As AI enters decision-making fields such as finance, employment, and criminal justice, these algorithms can reinforce prejudices. The ability to build new and fairer systems of power without compromising large amounts of data is a realistic way for organizations to integrate AI into the world while doing things ethically and responsibly.
According to the experts, they stress that their approach does more than improve equity; they also debunk the myth that one cannot have both quality models and accurate bias. In the past, efforts aimed at addressing bias were perceived as a cost that could potentially reduce the performance of the model. However, this study showed that one can work towards achieving fairness alongside efforts to improve performance, and in fact, the two can complement each other if pursued with a right attitude.
The use of this method is a great step forward in the ongoing process of creating ethical AI. Such developments in artificial intelligence are crucial in ensuring that the societies are augmented by such technologies and that such an impact is well distributed and effective. MIT team work breezed through incredible tasks and highlighted what AI is capable of doing; not only in the advancement but also in eradicating bias, accuracy and ethical standards.
Source: https://news.mit.edu/2024/researchers-reduce-bias-ai-models-while-preserving-improving-accuracy-1211
Latest Stories:
Thoughtworks and AWS Unite to Drive Generative AI Innovation in Asia-Pacific
Ericsson AI Solution Elevates Mobily’s 5G Network in Saudi Arabia
Infortrend Boosts AI Training with High-Speed Hybrid Flash Storage